Feature Story
The Subset Seekers
Trying to predict whether a patient will become a severe or mild case has been one of the greatest challenges of the COVID-19 pandemic. Data scientists and clinicians used artificial intelligence to try and help make those predictions, but the process turned up some of its own surprises and opened new opportunities.
When the first COVID-19 patients came through the doors of Johns Hopkins Hospital in 2020, Dr. Brian Garibaldi felt a mix of emotions: concern for the safety of family, colleagues, himself; pride that his team was hitting the front lines running to understand a newly discovered disease; and a cautious tinge of excitement.
“There aren’t many times in our careers where you get to see a disease that you’ve never even heard of,” he recalled of those early days.
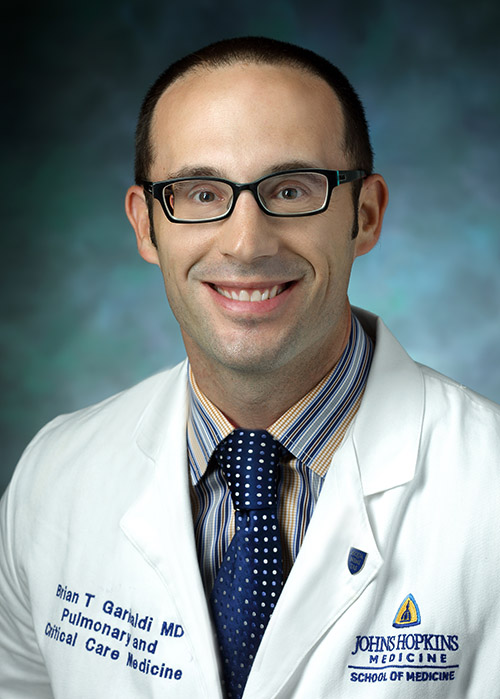
Credit: Johns Hopkins Medicine
Garibaldi directs the Johns Hopkins Biocontainment Unit in downtown Baltimore, one of 10 federally funded infectious disease treatment centers across the United States that had been preparing for the next viral outbreak since 2014, when Ebola struck West Africa. Clinicians, health care providers, researchers and infection control specialists had all self-selected to be on the front lines. Johns Hopkins was one of the first biocontainment units to activate when COVID-19 broke out in the U.S., and in those first three weeks in March 2020, the nation’s clinical eyes all turned to them.
Health care workers wanted to know what it was like to care for COVID-19 patients, to get the team’s clinical impressions so other health care centers could think about how to organize their resources and mobilize their care systems to learn about this novel virus as quickly as possible.
There was a feeling of tremendous responsibility upfront, Garibaldi said, having to pay close attention to everything they saw, and everything their patients told them. “We might make an observation that would lead to an important discovery, an important way of helping care for COVID patients down the road,” he said.
Within a few weeks, though, there were more patients than their unit could handle. People who’d never prepared to be front-line workers had to step in, providing support to those who had. The biocontainment unit had to integrate with the hospital’s intensive care unit — 24 beds that in normal times, Garibaldi said, would have maybe three or four patients needing mechanical ventilation. By April 2020 every bed had someone on a ventilator.
The connections between patient, symptom and disease trajectory — whether a person would become a mild or severe COVID-19 case — started to blur.
When asked about whether this or that parameter could predict the likelihood of someone needing a ventilator or extra care, Garibaldi couldn’t make ends meet. “To be honest,” he recalled responding, “we have so many patients at this point, I can’t remember what the relationship might be.”
Given the many patients who have followed an unlikely disease course based on their conditions, making a prediction would have been tough in any case. One of the first patients who arrived at Johns Hopkins, Garibaldi said, was a fit, active young man in his 30s. Yet he was bedridden in the hospital on a ventilator for 40 days before he recovered. To Garibaldi, that’s a striking example of how anyone could be severely affected by the virus. But it also underscored the difficulty in deciphering what disease course someone wheeled into the hospital will take.
People like Lucile Randon, a 117-year-old French nun who contracted the virus in mid-January 2021, for example, can take you by surprise. Blind, riding in a wheelchair and hailed as the second-oldest person in the world, Randon would without much debate be considered one of those most vulnerable to COVID-19. Yet three weeks after contracting the virus, she fully recovered, unfazed. According to the French newspaper Var-Matin, Randon hadn’t even realized she had COVID-19.
Because people were reacting to COVID-19 so differently, clinicians sensed there must be subtypes of patients, said Hannah Cowley, a data scientist at the Johns Hopkins Applied Physics Laboratory in Laurel, Maryland. “Some young people who are healthy succumbed to the disease, whereas some older patients with multiple comorbidities never did. It doesn’t make sense.”
Clinicians are struggling to predict who is going to do poorly, why and when. That makes it tough to make clinical decisions, and to have discussions with those patients’ families.
Cowley was part of a small team of data scientists from APL who stepped in to work with clinicians, including Garibaldi, to help make sense of it all. Leveraging their skills in machine learning with COVID-19 patient data collected by Johns Hopkins clinicians and hosted in a relatively new tool called the Precision Medicine Analytics Platform, or PMAP, the team aimed to identify COVID-19 patient subtypes and calculate what disease course was most probable for each.
“Clinicians are struggling to predict who is going to do poorly, why and when,” Cowley said. “That makes it tough to make clinical decisions, and to have discussions with those patients’ families.”
The team knew the most critical questions they needed to answer, although straightforward, were difficult: What if we could flag a patient who is likely to need a respirator or other form of supplementary oxygen, versus a person who will likely never go to the ICU?
What if we could administer medicine and care precisely, to meet each individual’s case?
Predicting Precisely
At its heart, precision medicine aims to do just that: develop care for patients tailored specifically to them as individuals rather than to them as the average person. It assumes people, through biostatistics, data science and a deep clinical understanding of biological mechanisms, can be divvied up into subtypes that vary in their risk to a disease or treatment because of underlying biological differences or other characteristics, from diet and exercise routines to family and medical history.
With big data and powerful machine-learning tools widely available today, precision medicine has gained traction in medical and political communities, such as the federal government’s Precision Medicine Initiative in 2015. But few of those initiatives have made the jump from scientific discovery to actual tools that can directly affect patient care.
There are many ways you can approach learning from clinical data, looking for patterns to indicate patients who might provide samples that are most informative, or for formulating new research questions, Garibaldi said.
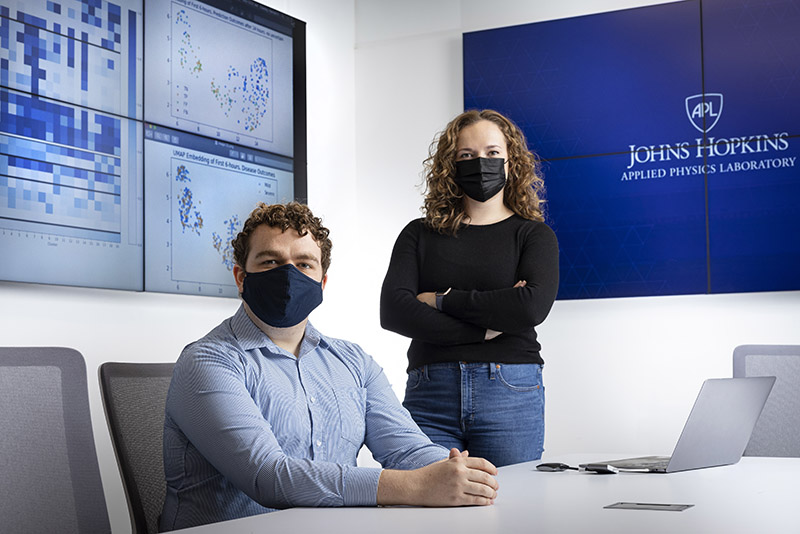
Credit: Johns Hopkins APL
In a paper published last September, he led a study to predict the trajectory of hospitalized patients with COVID-19. The research team linked the demographic characteristics, medical history, comorbidities, symptoms, vital signs, respiratory events, medications and laboratory results of more than 800 COVID-19 patients admitted to the Johns Hopkins Hospital network between March and April 2020 with whether they were predicted to develop severe conditions or death.
The model predicted outcomes for patients using just information collected at the time of hospital admission with 80‑85% accuracy at two, four and seven days after the patient was hospitalized.
The APL team, on the other hand, looked deeper. They aimed to combine people that seem to run the same disease course based on basic data about them, Cowley explained. “We only use variables or stats that you could easily get about a person.” Age, race, sex, ethnicity, self-reported comorbidities, COVID-19 symptoms, vital signs — such as pulse rate and blood oxygen saturation — and common laboratory blood tests.
People started to call the team “the subset seekers.”
Sussing Out the Subtypes
The APL team, led by APL electrical engineer Will Gray Roncal, first triaged data collected from 1,182 patients from March to August 2020. They created an algorithm to predict the most likely course a patient would take two weeks from the time they were admitted to the hospital, based on certain thresholds: If the probability of a mild outcome met or exceeded the mild threshold, the patient was considered mild (a negative result). If the probability of a severe outcome met or exceeded the severe threshold, they were considered a severe case (a positive result).
If the algorithm couldn’t make a prediction, it would collect data on the patient’s vitals and blood tests 6 hours later and try predicting again. If it still failed, it would try again 6 hours later. And again. And again. Every 6 hours for 48 hours.
Then they left the machine to cluster the same data on its own, looking for patterns that would be extremely difficult, if not impossible, for a human to identify — a process called unsupervised machine learning.
“It’s like a pattern-recognition robot, finding blobs of patients and grouping them together purely based on the data available,” explained Michael Robinette, another APL data scientist on the team.
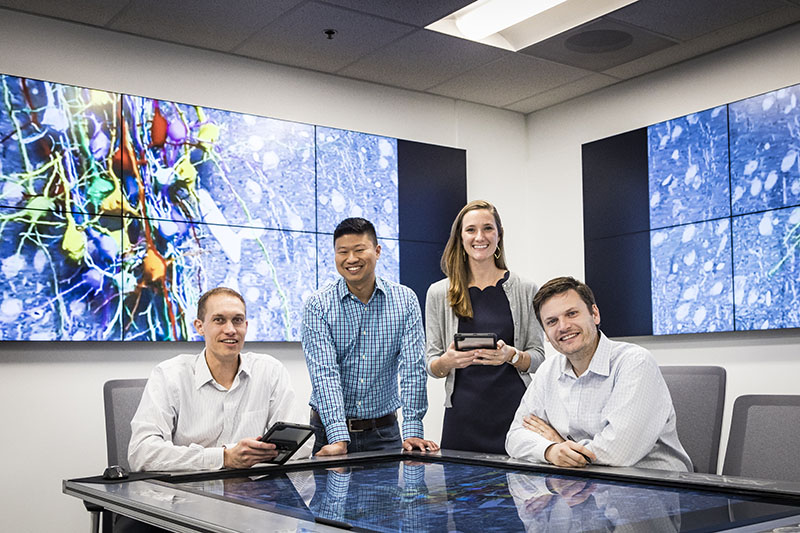
Credit: Johns Hopkins APL
Combining the methods, the team found patients fell into 20 clusters, divvied up by specific symptoms — muscle pain, chills, vomiting, loss of taste and smell, sore throat, fatigue — and comorbidities that included cardiovascular disease, hypertension and diabetes.
Some clusters were associated with a more mild disease course. In clusters 11 and 15, for example, 90‑92% of people followed a mild disease course. And oddly, those people tended to have fewer comorbidities but more severe symptoms.
“It’s possible it could be a fluke in the model,” Robinette said. Clinicians, however, offered the alternative possibility that those people may have very reactive immune systems, aggressively responding to the virus so that, at first, they have debilitating symptoms. But they ultimately end up being a mild case.
“That was definitely something that took me by surprise,” Robinette said.
However, of greatest interest (and most concern) were people in clusters 0, 3, 12, 18 and 19 — five clusters in which more than 5% of patients, a standard threshold of statistical significance, were predicted to follow a mild disease course but ended up becoming severe cases. They are the false-negative patients, and nobody is certain why.
“It’s not necessarily that our model is performing poorly,” Cowley said. “It’s that these people really are exceptional.”
Many people looked quite healthy from the outset. According to the data, few had comorbidities, such as cardiovascular disease or diabetes. Moreover, their COVID-19 symptoms, at least, tended to be headaches and, at worst, muscle pain and a cough. Even more unusual was their pulse oxygen levels (the amount of oxygen flowing in their blood). They all had fairly normal oxygen levels.
These patients look healthy, but clearly something makes them unhealthy. We know there is something different about these people. We just don’t know what it is.
By the model’s rules, and what we anecdotally and retrospectively know about COVID-19, these patients should turn out fine. But at some point, they take a 180-degree turn.
“We’re actually really frustrated by it,” Cowley said. “These patients look healthy, but clearly something makes them unhealthy. We know there is something different about these people. We just don’t know what it is.”
Robinette, although not a physician, surmised patients could be experiencing delayed responses, such as comorbidities that take effect after their algorithm has already made a judgment call. “Most patients are classified within 6 or 12 hours,” he said. A result with a patient having a worse outcome after a day or so, then, isn’t totally astonishing.
Clinicians are wary of results like this, Robinette added, but they’re not completely surprised. That’s especially because everything about this disease is new. “It’s something we’re very cautious about and looking for more clinical validation of,” Robinette said.
Garibaldi, however, found the anomalies fascinating. He figured a lot of new information and long-term value could be gleaned from those patients, particularly in generating new hypotheses. They could drive which research questions to ask, or which patients to investigate in the future.
“We can learn from those patients,” he said. “And hopefully, as we develop better ways of treating the complications of COVID and being able to better impact the disease trajectory, it can help us identify the right patients for the right treatment at the right time.”
That, he believes, is where the true promise of these techniques lies.
After the Terrible Fight
Nobody is certain how they would implement the prediction power of this machine-learning algorithm in hospitals, putting it directly into the hands of clinicians. Digital software installed on their computers? An app on their phones?
To make an actual medical tool requires a laundry list of tests, certifications and federal approvals. “It’s not easy,” Cowley said, and aside from the model’s result still needing rounds of peer review, there’s much room for it to improve. The team is still trying to understand what the differences among the 20 clusters mean, and they are considering collaborating with other institutions to access more datasets.
There’s also the question of whether the technique, at this point with millions already dead and efforts to vaccinate billions of people well underway, can make a splash in this pandemic, or if it’s a ripple with hopes of a splash when the next threat comes along.
Cowley is confident it can. “It’s great that we’re nearing the end of a terrible, terrible fight here,” she said, “but there are still plenty of people that need to be cared for.” With variants of the coronavirus now distributed globally, wreaking catastrophic havoc in places such as India, COVID-19 cases worldwide climbed for months after their precipitous drop in January 2021. They only recently started to fall again. As of May 10, the daily cases worldwide dropped by 12% from one week earlier, falling from 5,492,658 to 4,809,520 confirmed cases, according to the World Health Organization.
The challenges, individuality and lessons of this pandemic will likely make a mark well into the future, regardless. “Clinicians are going to be researching this event for years to come,” Cowley added.
I think there’s hope that we will apply these techniques on a much broader scale and continue to learn about other diseases much in the way that we’ve learned so much about COVID in just the last year.
Garibaldi expressed a similar sentiment, pointing out that a prediction model that can portend a patient’s disease progression with COVID-19 should be applicable to other acute diseases that lead to hospitalizations, such as pneumonia.
“I think there’s hope that we will apply these techniques on a much broader scale and continue to learn about other diseases much in the way that we’ve learned so much about COVID in just the last year,” he said.
But if nothing else, he added, the model, the pandemic and COVID-19 have accelerated everyone’s appreciation and understanding of the realized benefits of precision medicine, pushing it to the forefront much faster than it likely would have happened without the pandemic.
“I think it’s going to be a lot easier to convince health care researchers and leaders to recognize the power of this approach to big data,” Garibaldi said. “I think that’s going to last long after the pandemic.”
This work is based out of APL’s Intelligent Systems Center. To read more about the ISC and its projects, visit https://www.jhuapl.edu/isc/.
